Enhancing Urban Resilience Against Liquefaction Through AI-Based Soil Stability Predictions
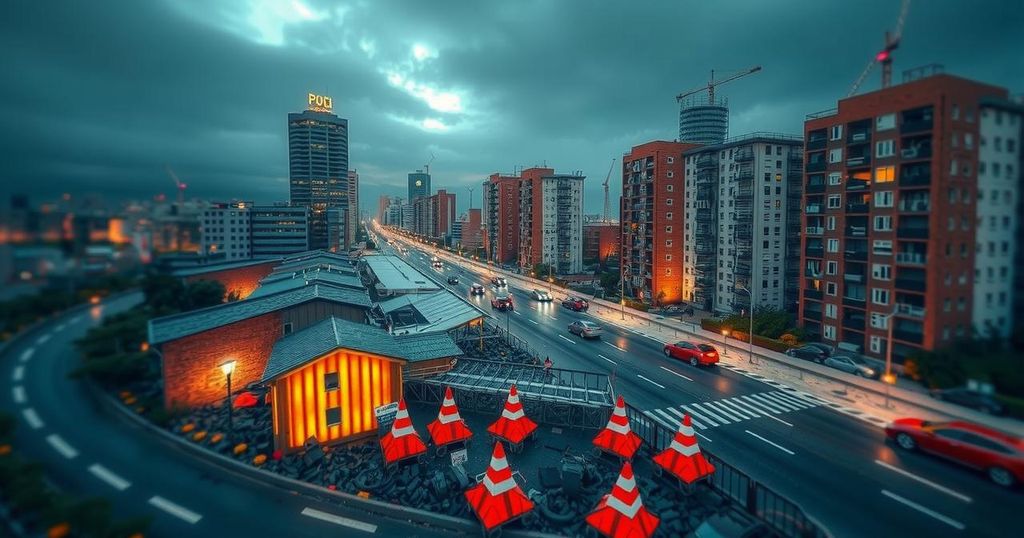
Researchers at Shibaura Institute of Technology have developed a machine learning model that predicts soil behavior during earthquakes, addressing the issue of liquefaction. Utilizing data from Setagaya, Tokyo, this model produces 3D maps of soil stability that assist city planners in identifying safe construction sites. The study demonstrates a 20% improvement in prediction accuracy compared to traditional methods, emphasizing the importance of data-driven strategies for enhancing urban resilience.
To enhance urban resilience in earthquake-prone regions, a group of researchers from Shibaura Institute of Technology in Japan has developed an innovative machine learning model aimed at predicting soil behavior during seismic events. This advancement addresses the critical issue of soil liquefaction, a phenomenon where saturated soil loses strength under shaking, leading to structural failures and significant damage. The model utilizes artificial neural networks and ensemble learning techniques to create three-dimensional maps portraying the depth of stable soil layers, crucial for identifying safe construction sites. Utilizing data from 433 locations in Setagaya, Tokyo, this approach provides vital insights for disaster management and urban planning, thereby contributing to the creation of safer cities. The study, published on 8 October 2024 in Smart Cities, presents a substantial improvement over traditional manual testing methods, with a reported 20% enhancement in prediction accuracy through advanced data training techniques. This innovative mapping tool is expected to facilitate better infrastructure planning and increase the overall safety and resilience of urban areas against liquefaction risks.
The phenomenon of soil liquefaction poses a significant risk to infrastructure in earthquake-prone areas, particularly in regions like Japan that experience frequent seismic activities. During major earthquakes, loosely packed, saturated soils can behave like a liquid, potentially leading to disastrous structural failures, as evidenced by past events such as the 2011 Tōhoku earthquake and the Christchurch earthquake. Given the expanding urban landscape, the need for enhanced predictive models for assessing soil stability is more critical than ever. Traditional soil testing methods are often insufficient, as they may not provide comprehensive data across vast areas. The introduction of machine learning techniques offers a promising alternative by allowing for more expansive data analysis and detailed soil behavior predictions, assisting city planners in making informed decisions about urban development and disaster preparedness.
In summary, the development of machine learning models to predict soil behavior represents a significant advancement in civil engineering and urban planning, particularly in earthquake-prone areas. By providing detailed insights into soil stability and liquefaction risks, these models will enable better planning and construction practices, ultimately contributing to the resilience and safety of urban environments. As cities continue to grow and face increasing natural disaster threats, integrating advanced technologies into urban planning will be paramount for fostering safer and smarter cities.
Original Source: www.preventionweb.net